In the lightning-fast, ever-evolving landscape of artificial intelligence (AI), the NVIDIA DGX GH200 emerges as a beacon of innovation. This powerhouse of a system, designed with the most demanding AI workloads in mind, is a complete solution set to revolutionize how enterprises approach Generative AI. NVIDIA has new details showing how the GH200 comes together and offers a peak at what AI performance looks like with this latest-generation GPU tech.
In the lightning-fast, ever-evolving landscape of artificial intelligence (AI), the NVIDIA DGX GH200 emerges as a beacon of innovation. This powerhouse of a system, designed with the most demanding AI workloads in mind, is a complete solution set to revolutionize how enterprises approach Generative AI. NVIDIA has new details showing how the GH200 comes together and offers a peak at what AI performance looks like with this latest-generation GPU tech.
NVIDIA DGX GH200: A Complete Solution
The DGX GH200 is not just a fancy piece of rack hardware; it’s a comprehensive solution that combines high-performance computing (HPC) with AI. It’s designed to handle the most complex AI workloads, offering a level of performance that is truly unparalleled.
The DGX GH200 pulls together a complete hardware stack, including the NVIDIA GH200 Grace Hopper Superchip, NVIDIA NVLink-C2C, NVIDIA NVLink Switch System, and NVIDIA Quantum-2 InfiniBand, into one system. NVIDIA is backing all of this with an optimized software stack specifically designed to accelerate the development of models.
Specification | Details |
---|---|
GPU | Hopper 96 GB HBM3, 4 TB/s |
CPU | 72 Core Arm Neoverse V2 |
CPU Memory | Up to 480 GB LPDDR5 at up to 500 GB/s, 4x more energy efficient than DDR5 |
CPU-to-GPU | NVLink-C2C 900 GB/s bi-directional coherent link, 5x more energy efficient than PCIe Gen5 |
GPU-to-GPU | NVLink 900 GB/s bi-directional |
High-speed I/O | 4x PCIe Gen5 x16 at up to 512 GB/s |
TDP | Configurable from 450W to 1000W |
Extended GPU Memory
The NVIDIA Grace Hopper Superchip, equipped with its Extended GPU Memory (EGM) feature, is designed to handle applications with massive memory footprints, larger than the capacity of its own HBM3 and LPDDR5X memory subsystems. This feature allows GPUs to access up to 144TBs of memory from all CPUs and GPUs in the system, with data loads, stores, and atomic operations possible at LPDDR5X speeds. The EGM can be used with standard MAGNUM IO libraries and can be accessed by the CPU and other GPUs through NVIDIA NVLink and NVLink-C2C connections.
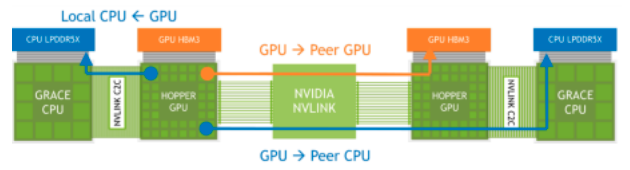
NVLink Memory Accesses Across Connected Grace Hopper Superchips
NVIDIA says that the Extended GPU Memory (EGM) feature on the NVIDIA Grace Hopper Superchip significantly enhances the training of Large Language Models (LLMs) by providing a vast memory capacity. This is because LLMs typically require tremendous amounts of memory to store their parameters, computations, and manage training datasets.
Having the ability to access up to 144TB of memory from all CPUs and GPUs in the system, models can be trained more efficiently and effectively. A large memory capability should lead to higher performance, more complex models, and the ability to work with larger, more detailed datasets, thereby potentially improving the accuracy and utility of these models.
NVLink Switch System
As the demands of Large Language Models (LLMs) continue to push the boundaries of network management, NVIDIA’s NVLink Switch System remains a robust solution. Harnessing the power of fourth-generation NVLink technology and third-generation NVSwitch architecture, this system delivers high-bandwidth, low-latency connectivity to an impressive 256 NVIDIA Grace Hopper Superchips within the DGX GH200 system. The result is a staggering 25.6 Tbps of full-duplex bandwidth, marking a substantial leap in data transfer speeds.
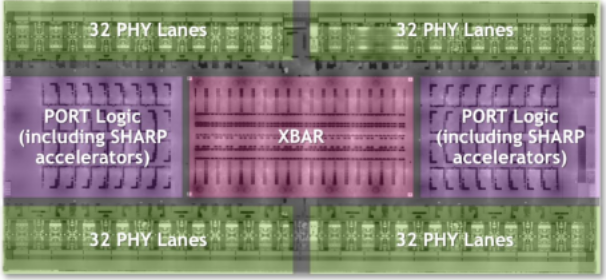
DGX GH200 Supercomputer NVSwitch 4th gen NVLink Logic Overview
In the DGX GH200 system, every GPU is essentially a nosy neighbor, being able to poke into the HBM3 and LPDDR5X memory of its peers on the NVLink network. Coupled with the NVIDIA Magnum IO acceleration libraries, this “nosy neighborhood” optimizes GPU communications, efficiently scales up, and doubles down on the effective network bandwidth. So, while your LLM training is being supercharged and communication overheads are taking a hike, AI operations are getting a turbo boost.
The NVIDIA NVLink Switch System in the DGX GH200 is capable of significantly enhancing the training of models like LLMs by facilitating high-bandwidth, low-latency connectivity between a large number of GPUs. This leads to faster and more efficient data sharing between GPUs, thereby improving the model’s training speed and efficiency. Moreover, every GPU’s ability to access peer memory from other Superchips on the NVLink network increases the available memory, which is critical for large parameter LLMs.
While the impressive performance of the Grace Hopper Superchips is indisputably a game-changer in the realm of AI computations, the real magic of this system happens in the NVLink, where high-bandwidth, low-latency connectivity across numerous GPUs takes data sharing and efficiency to an entirely new level.
DGX GH200 System Architecture
The architecture of the DGX GH200 supercomputer is complex, yet meticulously designed. Consisting of 256 GH200 Grace Hopper compute trays and an NVLink Switch System that forms a two-level NVLink fat tree. Each compute tray houses a GH200 Grace Hopper Superchip, networking components, a management system/BMC, and SSDs for data storage and operating system execution.
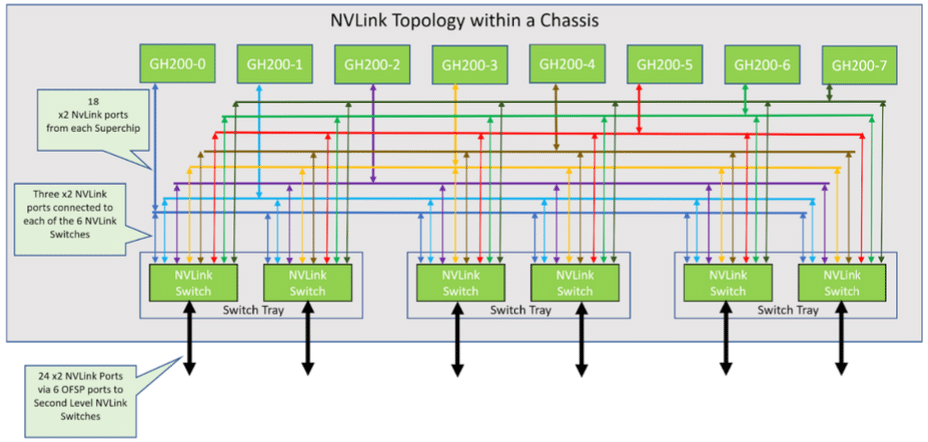
NVLink topology in 8-GraceHopper Superchip Chassis
Category | Details |
---|---|
CPU/GPU | 1x NVIDIA Grace Hopper Superchip with NVLink-C2C |
GPU/GPU | 18x NVLink fourth-generation ports |
Networking | 1x NVIDIA ConnectX-7 with OSFP: > NDR400 InfiniBand Compute Network 1x Dual port NVIDIA BlueField-3 with 2x QSFP112 or 1x Dual port NVIDIA ConnectX-7 with 2x QSFP112: > 200 GbE In-band Ethernet network > NDR200 IB storage network Out of Band Network: > 1 GbE RJ45 |
Storage | Data Drive: 2x 4 TB (U.2 NVMe SSDs) SW RAID 0 OS Drive: 2x 2 TB (M.2 NVMe SSDs) SW RAID 1 |
In this setup, eight compute trays are linked to three first-level NVLink NVSwitch trays to establish a single 8-GPU chassis. Every NVLink Switch tray possesses two NVSwitch ASICs that connect to the compute trays through a custom blind mate cable cartridge and to the second-level NVLink Switches via LinkX cables.
The resulting system comprises 36 second-level NVLink Switches that connect 32 chassis to form the comprehensive NVIDIA DGX GH200 supercomputer. For further information, refer to Table 2 for specifications of the compute tray with Grace Hopper Superchip, and Table 3 for NVLink Switch specifications.
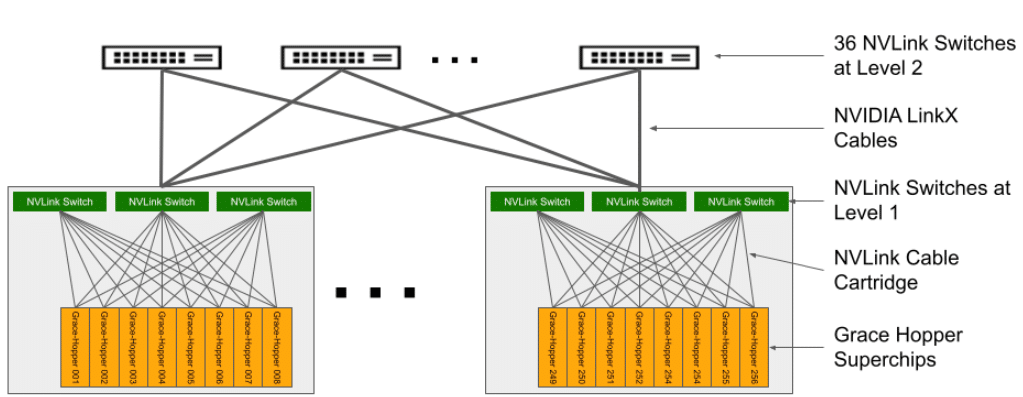
DGX GH200 NVLink Topology
Network Architecture of the DGX GH200
The NVIDIA DGX GH200 system incorporates four sophisticated network architectures to provide cutting-edge computational and storage solutions. Firstly, a Compute InfiniBand Fabric, constructed from NVIDIA ConnectX-7 and Quantum-2 switches, forms a rail-optimized, full-fat tree NDR400 InfiniBand fabric, enabling seamless connectivity between multiple DGX GH200 units.
Secondly, the Storage Fabric, driven by the NVIDIA BlueField-3 Data Processing Unit (DPU), delivers high-performance storage via a QSFP112 port. This establishes a dedicated, customizable storage network that skillfully prevents traffic congestion.
The in-band Management Fabric serves as the third architecture, connecting all system management services and facilitating access to storage pools, in-system services such as Slurm and Kubernetes, and external services like the NVIDIA GPU Cloud.
Lastly, the out-of-band Management Fabric, operating at 1GbE, oversees essential out-of-band management for the Grace Hopper superchips, BlueField-3 DPU, and NVLink switches through the Baseboard Management Controller (BMC), optimizing operations and preventing conflicts with other services.
Unleashing the Power of AI – NVIDIA DGX GH200 Software Stack
The DGX GH200 has all the raw power developers could want; it is much more than just a fancy super-computer. It’s about harnessing that power to drive AI forward. Undoubtedly, the software stack that comes bundled with the DGX GH200 is one of its standout features.
This comprehensive solution comprises several optimized SDKs, libraries, and tools designed to fully harness the hardware’s capabilities, ensuring efficient application scaling and improved performance. However, the breadth and depth of the DGX GH200’s software stack merits more than a passing mention, make sure to check out NVIDIA’s Whitepaper on the topic for a deep dive into the software stack.
Storage Requirements of the DGX GH200
To fully leverage the DGX GH200 system’s capabilities, it is crucial to pair it with a balanced, high-performance storage system. Each GH200 system has the capacity to read or write data at speeds of up to 25 GB/s across the NDR200 interface. For a 256 Grace Hopper DGX GH200 configuration, NVIDIA suggests an aggregate storage performance of 450GB/s to maximize read-throughput.
The need to fuel AI projects, and the underlying GPUs, with appropriate storage is the most popular tradeshow circuit talk of the summer. Quite literally every show we’ve been to has some segment of their keynote dedicated to AI workflows and storage. It remains to be seen, however, how much of this talk is just re-positioning existing storage products and how much of it leads to meaningful enhancements for AI storage. At the moment it’s too early to tell, but we’re hearing many rumblings from storage vendors that have the potential to lead to meaningful change for AI workloads.
One Hurdle Jumped, More to Follow
While the DGX GH200 streamlines the hardware design aspect of AI development, it is important to recognize that in the field of Generative AI there are other considerable challenges; the generation of training data.
The development of a Generative AI model necessitates an immense volume of high-quality data. But data, in its raw form, is not immediately usable. It requires extensive collection, cleaning, and labeling efforts to make it suitable for training AI models.
Data collection is the initial step, and it involves sourcing and accumulating vast amounts of relevant information, which can often be time-consuming and expensive. Next comes the data cleaning process, which requires meticulous attention to detail to identify and correct errors, handle missing entries, and eliminate any irrelevant or redundant data. Finally, the task of data labeling, an essential stage in supervised learning, involves classifying each data point so the AI can understand and learn from it.
Quality of the training data is paramount. Dirty, poor quality or biased data can lead to inaccurate predictions and flawed decision-making by the AI. There is still a need for human expertise and huge effort is necessary to ensure the data used in training is both plentiful and of the highest quality.
These processes are non-trivial, requiring significant resources, both human and capital, including specialized knowledge of the training data, underscoring the complexity of AI development beyond the hardware. Some of this is being addressed with projects like NeMo Guardrails which is designed to keep Generative AI accurate and safe.
Closing Thoughts
The NVIDIA DGX GH200 is a complete solution positioned to redefine the AI landscape. With its unparalleled performance and advanced capabilities, it’s a game-changer set to drive the future of AI. Whether you’re an AI researcher looking to push the boundaries of what’s possible or a business looking to leverage the power of AI, the DGX GH200 is a tool that can help you achieve your goals. It will be intriguing to observe how the generation of training data is addressed as raw compute power becomes more widespread. This aspect is frequently overlooked in discussions about hardware releases.
All things considered, it’s important to acknowledge the high cost of the DGX GH200 system. The DGX GH200 doesn’t come cheap and its premium price tag puts it squarely within the realm of the largest enterprises and the most well-funded AI companies (NVIDIA, hit me up, I want one), but for those entities that can afford it, the DGX GH200 represents a paradigm-shifting investment, one that has the potential to redefine the frontiers of AI development and application.
As more large enterprises adopt this technology and begin to create and deploy advanced AI solutions, it could lead to a broader democratization of AI technology. Innovations will hopefully trickle down into more cost-effective solutions, making AI more accessible to smaller firms. Cloud-based access to DGX GH200-like computational power is becoming more widely available, enabling smaller businesses to harness its capabilities on a pay-per-use basis. While the upfront cost may be high, the long-term influence of the DGX GH200 could ripple through the industry, helping to level the playing field for businesses of all sizes.
Engage with StorageReview
Newsletter | YouTube | Podcast iTunes/Spotify | Instagram | Twitter | TikTok | RSS Feed