Quantum Myriad is a modern, flexible SDS platform capable of handling a wide variety of workloads, including demanding AI tasks.
In 2023, Quantum launched a new, modern take on software-defined storage with Myriad. We completed a deep dive into Myriad late last year and were impressed with its extremely capable and resilient architecture. Myriad’s diverse feature set and protocol flexibility expand the scope of its use cases beyond enterprise file-sharing needs. And there is no application in more demand right now than AI.
AI is fundamentally transforming the enterprise landscape by bringing new insights to decision-making, automating complex processes, and creating new ways for companies to interact with customers and manage operations. Here are a few key areas where AI is making an impact:
Automation: AI automates routine, error-prone tasks like data entry and customer support with chatbots and even finds ways to optimize complex processes like supply chain management. This saves time and lets folks focus on more creative or strategic tasks.
Data Analysis: It digs through massive piles of data to find patterns and insights faster than any human could. This helps businesses make smarter decisions, predict market trends, and better understand their customers.
Personalization: Companies use AI to tailor their services and marketing to individual customers, like recommendation engines that learn what a user likes as seen on Netflix or Spotify.
Enhanced Security: AI is a big player in cybersecurity, helping to detect and respond to threats instantly. It’s always on guard, scanning for anything fishy.
Innovation: AI drives innovation by helping develop new products and services—from drugs to new materials—by simulating every aspect of a product’s design, look and feel, and maintenance. It can even predict outcomes before physical trials for medicines.
The Challenges of Scalability and Flexibility
Legacy storage systems often fail to scale efficiently, a capability crucial for AI applications that generate and process large data volumes. Traditional storage may require significant downtime or complex upgrades and networking configuration and allocation to increase capacity. This is not feasible in dynamic AI environments, which require rapid scalability, zero downtime, and model deployment without losing performance.
AI workloads also demand high throughput and low latency simultaneously. Legacy systems, usually equipped with mechanical drives and outdated networking, can’t meet these speed requirements, leading to bottlenecks that impede AI operations. Modern AI benefits dramatically from faster technologies like NVMe and GPU acceleration, and these are often incompatible with older systems.
AI applications require real-time data access and analysis from varied sources. Legacy storage, often siloed, hinders data integration and movement, limiting effective data analytics and machine learning. Legacy management tools also struggle with complex data governance and automated tiering necessary for AI.
Legacy storage maintenance and upgrades are also costly and inefficient for AI demands, including high operational costs for power, cooling, and space. Retrofitting old systems to support new technologies is economically unsustainable.
AI thrives on modern storage features like automated tiering, real-time analytics, and robust security measures like encryption. Legacy systems lack these critical capabilities, which are essential for protecting AI data and meeting regulatory standards.
Modernizing data management and storage infrastructures is vital for leveraging AI’s transformative potential. Next-generation storage solutions designed for AI complexities can significantly enhance performance, scalability, and cost efficiency, fostering innovative applications and business models.
Meet Quantum Myriad
Quantum Myriad is a high-performance, software-defined all-flash storage solution designed to meet the demands of modern applications, particularly those requiring high throughput and low latency. This is especially true for AI, data science, VFX, and animation. Myriad’s cloud-native architecture provides flexibility, ease of deployment, and automatic responses to system changes, whether on-premises or in a public cloud environment like AWS.
Myriad’s design zeroes in on delivering consistent low latency and high bandwidth. These qualities are absolute must-haves for applications that demand lightning-fast data processing and real-time performance.
Myriad’s architecture is highly flexible and effortlessly accommodates both small and large deployments. It is easy to start with a small system and then grow a Myriad cluster. As you add more nodes, it scales up linearly while still keeping things efficient and balanced.
Managing clusters with Myriad is simple. It comes packed with features like zero-click storage expansions and a user-friendly management portal, which help reduce the need for constant administrative attention. Myriad covers data integrity with a transactional key-value store spread across its nodes and error correction is managed across all available storage nodes.
Myriad comes with impressive networking support. With support for RDMA over Converged Ethernet (RoCE) and integration with existing network deployments via BGP routing, data can effortlessly flow in and out of the Myriad cluster while taking advantage of advanced networking capabilities.
Myriad is designed to be user-friendly and requires minimal steps to set up and run or add extra share locations. This makes it perfect for enterprises needing to adapt quickly to changing storage needs, particularly since the solution runs on standard, off-the-shelf servers. Plus, if you’re eyeing the cloud, Myriad plays nice with platforms like AWS so that you can extend beyond your on-premises setup.
Quantum Myriad Configuration as Tested
The Quantum Myriad tested was a base configuration of five nodes. Each node was equipped with ten 15TB SSDs, totaling a significant amount of fast-access storage across the cluster. This base setup allowed for substantial data storage capacity while maintaining the speed necessary for high I/O operations and quick data retrieval—all essential for real-time processing and AI computations. The storage nodes are specified and configured from SuperMicro and the NVMe drives are readily available from Samsung.
As tested, we used a Myriad platform configured in a 5 Node Cluster, each with the following key specifications:
- Quantum Myriad N1010 Storage Node with a Single 64-core AMD EPYC CPU
- 10 x 15.36TB NVMe TLC
- 2 x Dual Port 100GbE Ethernet ports
Integral to Myriad are the load balancer nodes deployed in a redundant pair cross-connected across all storage nodes. These are critical in managing the data traffic to and from the storage nodes. The pair of load balancers ensures that the network traffic is evenly distributed among the storage nodes, preventing any single node from becoming a bottleneck. Where simultaneous data access and processing are required, this is extremely important in environments where the speed and reliability of data access can significantly impact overall system performance.
Using multiple nodes and load balancers boosts performance and enhances the system’s reliability and fault tolerance. Distributing the storage and network load allows the system to continue operating efficiently even if one node encounters issues. This setup is essential for maintaining uptime and ensuring data integrity in critical business applications.
The base configuration is designed to be flexible and can be scaled up by adding more Storage Nodes as required. You can expand your storage capacity by incorporating additional nodes, using denser NVMe drives, or both. For instance, you might add a new Storage Node equipped with 30TB drives to a system currently using 15TB drives, or you could upgrade to denser drive modules within the existing footprint. This scalability is essential for businesses that expect to see growth in their data needs or experience variable data usage patterns.
Comino GPU Load Gen Servers
To exercise the Myriad system and generate our benchmarks, we used a pair of Comino Grando systems. The Comino Grando systems are high-performance, liquid-cooled setups designed specifically to maximize GPU efficiency and stability under load. They are particularly suitable for intense computational tasks like those encountered in AI, data analytics, and graphics-intensive applications. Here’s a summary of the key aspects that we configured for this testing:
Grando Server | Grando Workstation | |
---|---|---|
CPU | Threadripper Pro W5995WX | Threadripper Pro 3975WX |
Ram | 512GB RAM | 512GB RAM |
GPU | 2X NVIDIA A100 | 2X NVIDIA A100 |
NIC | 4x NVIDIA ConnectX 6 200G EN/IB | 4x NVIDIA ConnectX 6 200G EN/IB |
PSU | 4x 1600w PSUs | 3x 1000 SFX-L PSU |
Storage | 2TB NVMe | 2TB NVMe |
The Comino Grando leverages a sophisticated liquid cooling system for the processor and GPUs, which includes drip-free connections and a large water distribution block that efficiently manages coolant flow to maintain performance even under strenuous loads. This setup enhances performance and minimizes noise.
Using the nvidia-smi top -mp
command displays the GPU and NIC mapping in our system and the paths the data must take. Here is the legend:
X = Self SYS = Connection traversing PCIe as well as the SMP interconnect between NUMA nodes (e.g., QPI/UPI) NODE = Connection traversing PCIe as well as the interconnect between PCIe Host Bridges within a NUMA node PHB = Connection traversing PCIe as well as a PCIe Host Bridge (typically the CPU) PXB = Connection traversing multiple PCIe bridges (without traversing the PCIe Host Bridge) PIX = Connection traversing at most a single PCIe bridge
From here, we can tell that we would not want to use GPU1 with NIC4 and NIC5 for optimal performance, though this plays a limited role in our synthetic testing.
Quantum Myriad AI Testing
We conducted a technical analysis to evaluate the performance of the Quantum Myriad storage cluster and its impact on real-world AI workloads. Our analysis focused on the cluster’s ability to optimize resource utilization and scale effectively. Throughout this testing, we employed high-end Comino rack workstations equipped with NVIDIA ConnectX-6 200GbE NICs and dual NVIDIA A100 GPUs. These are crucial as they represent a robust testing environment similar to what might be employed in large-scale AI projects.
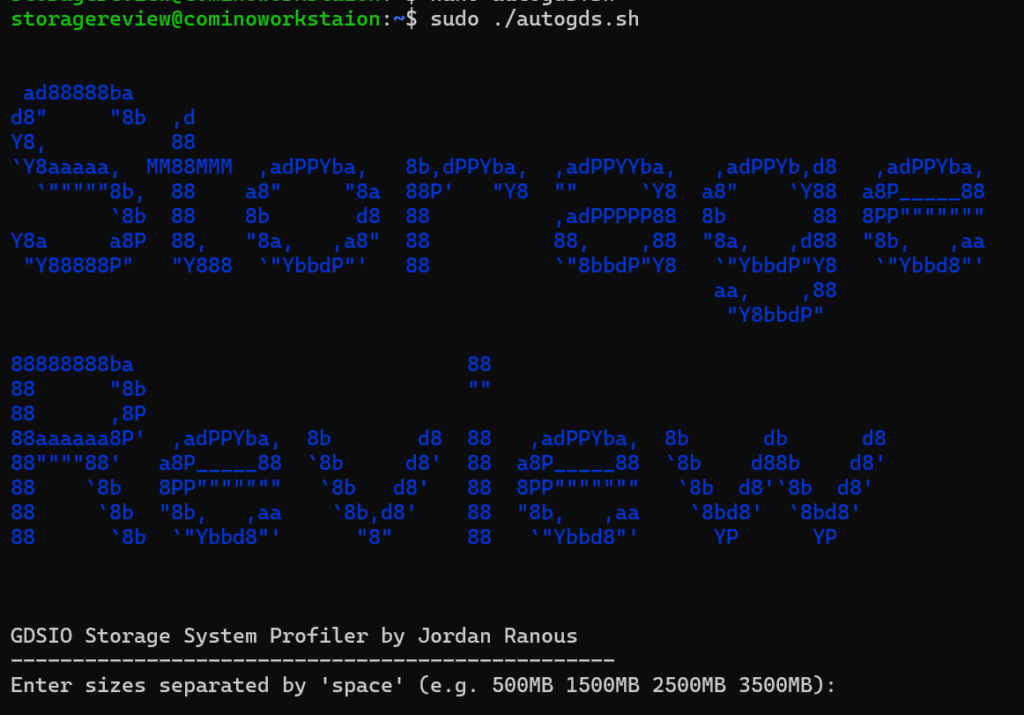
We leveraged a simple shell script to help create GDS test scripts and parse the output. ASCII Art for Style Points
The primary objective of these tests was to assess the Quantum Myriad cluster’s capability to handle intensive IO operations and how well it can accommodate throughput from high-capacity GPUs, which are critical for AI computations. Given that AI workloads heavily depend on the rapid processing of data sets, the ability of a storage solution to deliver data at speeds that match GPU processing capabilities directly impacts overall system efficiency and performance.
Our primary tool for testing here was NVIDIA’s GPUDirect Storage I/O (GDSIO). GPUDirect is a pivotal technology designed to enhance data transfer efficiencies between storage systems and GPUs, streamlining workflows that are critical in high-performance computing, artificial intelligence, and big data analytics.
This technology enables direct memory access (DMA) from storage directly to GPU memory, in effect bypassing the CPU. This eliminates unnecessary data copies, which reduces latency and improves throughput. GDSIO is the synthetic implementation of GPUDirect and is particularly representative of applications that require rapid processing of large datasets. This includes machine learning model training or real-time data analysis. It also provides profiling and tuning feedback for storage and networking infrastructure.
In the context of storage benchmarking, GDSIO plays a crucial role in accurately assessing the performance of storage solutions in environments heavily utilizing GPUs. By providing a more direct path for data transfer, GDSIO allows for benchmarking to measure the true potential of storage systems in supporting GPU-accelerated applications.
AI Share Configuration
When logging into Myriad, the user is presented with a dashboard that shows a high-level overview of the cluster’s current performance and specifications. Users can easily view telemetry data like IOPS, throughput, latency, and usage.
Mousing over any of the charts provides highly detailed performance information.
Looking at the File System screen, the currently configured mount points for shares are intuitively viewable.
The Nodes page is interesting, it shows the physical map of the cluster, controller, and load balancers, along with port activity and NVMe drives.
Clicking on any of the drives shows the status as reported by the host.
Heading to Shares, users can easily configure the shares as needed and get instructions for mounting them in various operating systems.
We worked with Quantum to set up a dedicated NFS share for our testing. These were mounted at /mnt/myriad/
This was achieved using the user-friendly Myriad UI, which offers a straightforward point-and-click configuration setup. During the testing period, the SMB option was in Early Access, while NFS remained the preferred protocol for our Linux-based workload machines.
Our NFS mount point was configured for 500TB of space, but you could use whatever you need by expanding the file system. You can freely overprovision storage with no penalties, and there is no hard limit to sizing. This becomes very interesting when you consider Myriad’s data compression, which essentially reduces the footprint of data on the NVMe SSDs.
With one mount point per host, each GPU has its own sub-folder, using its own NIC to avoid an NFSv4 limitation.
Quantum Myriad AI Results and Analysis
First, let’s look at the overall performance of one of our loadgen runs. This sample of one GPU’s perspective of the storage represents the performance we could see across all nodes/GPUs.
IO Type | IO Size (KiB) | Throughput (GiB/sec) | Avg Latency (usecs) | Operations |
---|---|---|---|---|
RANDWRITE | 1024 | 2.57 | 10,087.74 | 78,820 |
RANDREAD | 1024 | 6.92 | 2,277.86 | 209,319 |
RANDWRITE | 4096 | 3.44 | 18,193.14 | 56,616 |
RANDREAD | 4096 | 3.64 | 6,481.70 | 73,715 |
RANDWRITE | 4 | 0.03 | 2,307.57 | 237,512 |
RANDREAD | 4 | 0.12 | 497.05 | 941,971 |
WRITE | 1024 | 2.79 | 5,609.64 | 94,017 |
READ | 1024 | 3.11 | 5,021.91 | 95,556 |
WRITE | 4096 | 2.77 | 22,551.26 | 31,716 |
READ | 4096 | 3.50 | 17,875.32 | 31,871 |
WRITE | 4 | 0.08 | 812.93 | 580,169 |
READ | 4 | 0.12 | 507.34 | 926,909 |
Test results reveal significant insights into Myriad’s performance across various IO operations and sizes. Some of our findings include:
- Small and Large Block Performance: The tests show a marked difference in throughput and latency when handling small (4 KiB) versus large (1024 KiB and 4096 KiB) block sizes. For instance, large block RANDREAD operations at 4096 KiB demonstrated the highest throughput of approximately 9.64 GiB/sec, with a relatively lower average latency of 6,481.70 microseconds. This indicates excellent performance for large-scale data processing tasks common in machine learning model training, where large data sets are frequently accessed.
- GPU Saturating Capability: The throughput achieved during large block tests, particularly for RANDREAD operations, suggests that the Myriad storage cluster is quite capable of backing the NVIDIA A100 GPUs in inference retrieval-type workloads and for offloading checkpoints to a central location during training workloads. Given that the A100 can handle massive amounts of data for deep learning, the high throughput rates are essential for ensuring that these GPUs are not left idling awaiting data, thereby maximizing computational efficiency.
- Low Block Size Handling: Conversely, when examining operations with 4 KiB blocks, we observed a dramatic increase in operations count and latency, with a significant drop in throughput. This scenario is critical for understanding performance in environments where multiple small file transactions occur, such as in online transaction processing systems or databases handling numerous small queries.
But Wait, There’s More!
Focusing on the 4K tests, things took an interesting turn as we loaded up Myriad with more GPUs. Due to the limitations of the mounting protocol, as discovered in the initial runs during the discovery phase, Myriad was behaving as intended but with a surprising twist. As we loaded up Myriad across all of the GPUs simultaneously, thanks to some scripting hacks, the results were impressive. Myriad delivered essentially the same performance to all nodes simultaneously.
4K File Size
Here is a compilation of five simultaneous runs of the 4K workload:
Node | IO Type | Throughput (MiB/sec) | Avg Latency (usecs) | Operations |
---|---|---|---|---|
1 | RANDREAD | 125.73 | 497.05 | 941,971 |
2 | RANDREAD | 121.29 | 506.67 | 907,642 |
3 | RANDREAD | 128.37 | 474.73 | 906,847 |
4 | RANDREAD | 122.93 | 487.88 | 966,441 |
Total Random Read | 498.31 | 491.58 | 3,722,901 | |
1 | RANDWRITE | 27.08 | 2,307.57 | 237,512 |
2 | RANDWRITE | 26.88 | 2,285.62 | 231,625 |
3 | RANDWRITE | 26.10 | 2,406.89 | 228,983 |
4 | RANDWRITE | 28.27 | 2,341.65 | 245,172 |
Total Random Write | 108.34 | 2,335.43 | 943,292 | |
1 | READ | 123.19 | 507.34 | 926,909 |
2 | READ | 125.69 | 511.23 | 900,136 |
3 | READ | 123.90 | 502.04 | 945,949 |
4 | READ | 123.77 | 502.36 | 948,850 |
Total Read | 496.54 | 505.74 | 3,721,844 | |
1 | WRITE | 76.87 | 812.93 | 580,169 |
2 | WRITE | 80.17 | 839.88 | 551,311 |
3 | WRITE | 78.62 | 783.24 | 556,060 |
4 | WRITE | 73.40 | 811.62 | 597,226 |
Total Write | 309.06 | 811.92 | 2,284,766 |
4MB File Size
Node | IO Type | Throughput (GiB/sec) | Avg Latency (usecs) | Operations |
---|---|---|---|---|
1 | RANDREAD | 3.44 | 6,481.70 | 73,715 |
2 | RANDREAD | 3.97 | 6802.17 | 75,689 |
3 | RANDREAD | 3.83 | 6498.16 | 73,277 |
4 | RANDREAD | 3.50 | 6,589.43 | 70,443 |
Total Random Read | 14.75 | 6,593 | 293,124 | |
1 | RANDWRITE | 3.44 | 18,193.14 | 56,616 |
2 | RANDWRITE | 3.4048 | 19090.38 | 54,725 |
3 | RANDWRITE | 3.4349 | 18125.25 | 56,277 |
4 | RANDWRITE | 3.5084 | 17018.30 | 54,397 |
Total Random Write | 13.78 | 18,107 | 222,015 | |
1 | READ | 3.50 | 17,875.32 | 31,871 |
2 | READ | 3.4388 | 17110.93 | 31,119 |
3 | READ | 3.5133 | 18124.53 | 31,096 |
4 | READ | 3.3035 | 17755.53 | 31,257 |
Total Read | 13.75 | 17,717 | 125,343 | |
1 | WRITE | 2.77 | 22,551.26 | 31,716 |
2 | WRITE | 2.8845 | 23674.69 | 33,017 |
3 | WRITE | 2.7008 | 22661.31 | 30,971 |
4 | WRITE | 2.7719 | 22798.83 | 29,519 |
Total Write | 11.13 | 22,922 | 125,223 |
Quantum Myriad’s unique storage architecture provides dual accessibility that benefits both GPU operations and concurrent user activities without performance loss. This is particularly effective in high-demand environments where simultaneous data access and processing are required, similar to a user community of AI and machine learning server and end-user analyst access. By supporting large data block accessibility for GPUs alongside other user operations, Myriad ensures efficient resource utilization and prevents bottlenecks. This is crucial for maintaining high operational speeds and data accuracy in applications such as real-time analytics and AI model training.
It is worth noting here that the party trick of Quantum Myriad is its ability to adeptly handle multiple data streams right up to the limitations of NFSv4, which can be hit easily under intense GPU loads. The system’s sophisticated data management capabilities prevent these limits from impacting overall Myriad platform performance, ensuring that high-demand GPU tasks do not slow down other operations on the same network. This feature is particularly beneficial for industries that require robust data processing capabilities without sacrificing the performance of concurrent tasks, supporting a seamless workflow for all users.
Real-World Implications and Scaling
Quantum Myriad has the potential to work with real-world applications and scaling scenarios easily. Its ability to handle large block sizes with high throughput and low latency benefits AI workloads, including training deep learning models where large datasets are processed in batches. High throughput ensures data feeds into the GPUs without delay, which is crucial for maintaining high utilization and efficient learning.
Another essential characteristic is scalability. The performance of the Quantum Myriad storage cluster in our tests suggests that it can efficiently support larger configurations. As the number of connected devices (e.g., additional GPUs or other high-performance computing units) increases, the storage system appears capable of maintaining high levels of data delivery without becoming a bottleneck.
The Quantum Myriad storage cluster’s performance during large block RANDREAD operations was especially notable during our tests. This capability is critical when considering the needs of modern AI and machine learning frameworks.
The RANDREAD test, with its significant throughput, showcases Myriad’s ability to quickly and efficiently retrieve large volumes of data. This becomes particularly important in the context of inference workloads, where the speed at which data can be accessed directly impacts the performance of AI models in production environments. Inference tasks, which often require rapid access to large datasets for real-time decision-making, benefit immensely from the high-speed data retrieval capabilities exhibited by the Myriad cluster. For example, in applications like real-time image recognition or complex decision engines driving automated systems, the ability to pull large blocks of data with minimal latency ensures that the inference engines can operate at peak efficiency without stalling for data.
During the testing phase, Myriad demonstrated robustness in handling checkpoint data during training workloads, which is equally important as the cluster’s performance during write operations. Training modern AI models, especially deep learning networks, involves iterative processes where checkpoints are crucial. These checkpoints, which represent the state of the model at a particular iteration, need to be saved periodically to ensure that progress isn’t lost and that models can be effectively fine-tuned without retraining from scratch. Myriad efficiently offloads large write operations to the storage cluster, reducing I/O time and allowing GPUs to focus on computation instead of data handling.
Myriad’s architecture ensures that as data demands scale, whether due to increased dataset sizes or more complex model training requirements, the system can scale accordingly without creating bottlenecks, downtime, or loss of user connectivity. This scalability is essential in an era where AI and machine learning workloads are rapidly evolving, requiring storage solutions that keep pace with current demands and are future-proofed against upcoming advancements in AI research and development.
Conclusion
Quantum Myriad storage cluster exhibits exceptional performance in managing diverse and demanding I/O operations. It is a versatile solution for traditional business workloads and cutting-edge AI applications. Thanks to its high throughput and low latency, Myriad’s capabilities extend beyond traditional data warehousing tasks that are crucial for maintaining smooth operations and efficient data retrieval.
In addition to these conventional uses, Myriad’s robust performance characteristics make it an ideal candidate for the more intensive demands of AI workflows. Here, the cluster excels in scenarios requiring rapid data access and high-speed processing, vital for training sophisticated machine learning models and running complex neural networks. The ability to quickly read and write large, shared volumes of data can increase GPU utilization and ensure that AI computations can be carried out without delay.
This comprehensive testing of the Myriad cluster serves as a crucial benchmark for understanding its scalability and performance in environments that combine traditional IT and business needs with the high demands of AI research and development. The results highlight Myriad’s technical prowess and its potential to facilitate high-stakes AI applications and machine learning projects, underscoring its adaptability and efficiency across a broad spectrum of computational tasks. Such capabilities confirm Myriad’s role as a foundational technology supporting enterprises as they navigate current needs and future technological landscapes.
This report is sponsored by Quantum. All views and opinions expressed in this report are based on our unbiased view of the product(s) under consideration.
Engage with StorageReview
Newsletter | YouTube | Podcast iTunes/Spotify | Instagram | Twitter | TikTok | RSS Feed