Solidigm P5336 SSDs unlock new research frontiers by supporting the data-intensive requirements of AI and machine learning algorithms at the edge.
In an era where scientific research is rapidly advancing to the edges of our technological capabilities, the significance of high-capacity storage has become increasingly prominent. Armed with a powerful deep-sky-object capture rig, a set of Solidigm P5336 61.44TB QLC SSDs, and our new favorite rugged Dell PowerEdge XR7620 server, we explore the need for robust, cost-effective storage to manage the rapidly exploding data requirements of edge-based AI-accelerated scientific research.
Edge Data Capture
In recent years, scientific and data computing has undergone a monumental shift, transitioning from traditional, centralized computing models to the more dynamic realm of edge computing. This shift is not just a change in computing preferences but a response to modern data processing exploration’s evolving needs and complexities.
At its core, edge computing refers to processing data near the location where it is generated, as opposed to relying on a centralized data-processing warehouse. This shift is increasingly relevant in fields where real-time data processing and decision-making are crucial. Edge computing is compelling in scientific research, especially in disciplines that require rapid data collection and analysis.
The Factors Driving Edge Computing
Several factors drive the move toward edge computing in scientific research. Firstly, the sheer volume of data generated by modern scientific experiments is staggering. Traditional data processing methods, which involve transmitting massive datasets to a central server for analysis, are becoming impractical and time-consuming.
Secondly, the need for real-time analysis is more pronounced than ever. In many research scenarios, the time taken to transfer data for processing can render it outdated, making immediate, on-site analysis essential.
Lastly, more sophisticated data collection technologies have necessitated the development of equally sophisticated data processing capabilities. Edge computing answers this need by bringing powerful computing capabilities closer to data sources, thereby enhancing the efficiency and effectiveness of scientific research.
Scientific research, our edge computing focus for this article, is particularly interested in keeping as much raw data collected by modern, sophisticated sensors as possible. Real-time monitoring and analysis of the captured data using accelerators like the NVIDIA L4 at the edge provides summaries. Still, there is no replacement for capturing and preserving all data for future, more profound analysis. This is where the ultra-dense Solidigm QLC SSDs come in.
The Setup
Astrophotography, the practice of capturing images of celestial bodies and large areas of the night sky, is a prime example of a field that significantly benefits from edge computing. Traditionally, astrophotography is a discipline of patience, requiring long exposure times and significant post-processing of images to extract meaningful data. In the past, we looked at accelerating the process with a NUC cluster. Now, it’s time to take it to the next level.
The Edge Server
We used the ruggedized Dell PowerEdge XR7620 as the core server platform at the edge. These optimized servers are short-depth, dual-socket in a compact form factor, and offer acceleration-focused solutions. Unlike the typical edge servers, the XR7620 server addresses the rapid maturation of AI/ML with support for the most demanding workloads, including industrial automation, video, point-of-sale analytics, AI inferencing, and edge point-device aggregation.
Dell PowerEdge XR7620 Key Specifications
For a complete list of specifications, check out our full review here: Dell PowerEdge XR7620.
Feature | Technical Specifications |
Processor | Two 4th Generation Intel® Xeon® Scalable processors with up to 32 cores per processor |
Memory | 16 DDR5 DIMM slots, supports RDIMM 1 TB max, speeds up to 4800 MT/s. Supports registered ECC DDR5 DIMMs only |
Drive Bays | Front bays: Up to 4 x 2.5-inch SAS/SATA/NVMe SSD drives, 61.44 TB max, up to 8 x E3.S NVMe direct drives, 51.2 TB max |
This Dell PowerEdge server isn’t just any piece of tech. It’s built to withstand the harshest conditions the wild has to offer. Think sub-zero temperatures, howling winds, and the isolation that makes the word “remote” seem an understatement. But despite the odds, it proved to be capable and unyielding, powering the research with the might of its state-of-the-art processors and a monstrous capacity for data analysis.
Having a ruggedized server removes the pressure of keeping the server safe and warm. It’s not just the staging; it is also essential that the server can withstand the teeth-rattling drive from a secure location to a cold, isolated spot in the middle of nowhere.
The Telescope
For this test, we chose a location along the Great Lakes, within the heart of a remote wilderness, far removed from the invasive glow of city lights. The centerpiece of our astrophotography rig is the Celestron Nexstar 11-inch telescope. With an F/1.9 aperture and a 540mm focal length, this telescope is ideal for astrophotography in low-light conditions, offering remarkable detail for deep-sky exploration. In the profound stillness of the wilderness, this telescope stands as a sentinel, its lens trained on the heavens, ready to capture the celestial spectacle.
The One-Shot Camera
Attached to the Nexstar is the ZWO ASI6200MC Pro One Shot Color Camera. Engineered for the sole purpose of astrophotography, this camera can render high-resolution, color-rich images of astronomical objects. The choice of a one-shot color camera simplifies the imaging process, capturing full-color images in a single exposure without needing additional filters. This feature is invaluable in the remote wilderness, where simplicity and efficiency are paramount.
Specification | Detail |
---|---|
Sensor | SONY IMX455 CMOS |
Size | Full Frame |
Resolution | 62 Mega Pixel 9576×6388 |
Pixel Size | 3.76μm |
Bayer Pattern | RGGB |
DDR3 Buffer | 256MB |
Interface | USB3.0/USB2.0 |
The ZWO ASI6200MC Pro is a purpose-designed astrophotography camera equipped with a SONY IMX455 CMOS sensor, offering an impressive 62 Mega Pixel resolution across a full-frame sensor. It boasts a pixel size of 3.76μm, enabling detailed and expansive celestial captures with a maximum frame rate of 3.51FPS at full resolution.
The camera has an integrated cooling system — a regulated two-stage thermal electric cooler — to lower the temperature of the sensor to ensure optimal performance by maintaining a temperature 30°C-35°C below ambient levels, reducing electronic noise for more precise images. With features like a rolling shutter, a wide exposure range, and a substantial 256MB DDR3 buffer, this camera is designed to deliver exceptional quality images for both amateur and professional astronomers alike.
Maintaining a reliable data connection in the remote wilderness is not as challenging today with Starlink. This satellite-based internet service provides high-speed data connectivity, essential for transmitting data and receiving real-time updates, but with considerable bandwidth limitation for sending massive data sets back to the lab.
The High-Capacity Storage
Preserving every sub-frame in astrophotography is vital for researchers as it unlocks a wealth of information essential for advancing astronomical knowledge. Each sub-frame can capture incremental variations and nuances in celestial phenomena, which is crucial for detailed analysis and understanding. This practice enhances image quality through noise reduction and ensures data reliability by providing redundancy for verification and aiding in error correction and calibration.
Specification | Solidigm D5-P5336 7.68TB |
---|---|
Capacity | 7.68TB |
Sequential Read/Write | Up to 6.8GB/s Read / 1.8GB/s Write |
Random 4K Read/16K Write IOPS | Up to 770k IOPS Read / 17.9k IOPS Write |
Drive Writes Per Day (DWPD) | 0.42 DWPD with 16K R/W |
Warranty | 5 Years |
Additionally, we employ the use of the 61.44TB Solidigm D5-P5336 Drive
Specification | Solidigm D5-P5336 61.44TB |
---|---|
Capacity | 61.44TB |
Sequential Read/Write | Up to 7GB/s Read / 3GB/s Write |
Random 4K Read/16K Write IOPS | Up to 1M IOPS Read / 42.6k IOPS Write |
Drive Writes Per Day (DWPD) | 0.58 DWPD with 16K R/W |
Warranty | 5 Years |
Our primary interest is having a comprehensive dataset that enables the application of advanced computational techniques, like machine learning and AI, for uncovering patterns and insights that may be missed in manual analysis or traditional methods. Keeping these sub-frames also future-proofs the research, allowing for reprocessing with evolving technologies. It is also a historical record for long-term study and collaborative efforts, making it an invaluable resource.
StorageReview’s Innovative Approach
We have pushed the envelope covering edge computing and its importance—and not just for typical markets like industrial and retail use cases. Taking our kit to remote locations and documenting all phases of astrophotography image captures and compilations helps us understand how AI benefits us in many different aspects of life. You may recall our Extreme Edge review from last year, where we set up our rig in the desert to capture a night sky without worrying about artificial light affecting our images.
In the quest to push the boundaries of astrophotography, particularly at the edge where high-capacity storage and computational efficiency are paramount, a novel approach to image deconvolution is revolutionizing our ability to capture the cosmos with unprecedented clarity. To accomplish this goal, we introduced a groundbreaking convolutional neural network (CNN) architecture that significantly reduces the artifacts traditionally associated with image deconvolution processes.
The core challenge in astrophotography lies in combating the distortions introduced by atmospheric interference, mount and guiding errors, and the limitations of observational equipment. Adaptive optics have mitigated these issues, but their high cost and complexity leave many observatories in the lurch. Image deconvolution, the process of estimating and reversing the effects of the point spread function (PSF) to clarify images, is a critical tool in the astronomer’s arsenal. However, traditional algorithms like Richardson-Lucy and statistical deconvolution often introduce additional artifacts, detracting from the image’s fidelity.
Enter the innovative solution proposed for collaboration with Vikramaditya R. Chandra: a tailored CNN architecture explicitly designed for astronomical image restoration. This architecture not only estimates the PSF with remarkable accuracy but also applies a Richardson-Lucy deconvolution algorithm enhanced by deep learning techniques to minimize the introduction of artifacts. Our research demonstrates superior performance over existing methodologies by training this model on images we captured and from the Hubble Legacy Archive, presenting a clear path towards artifact-free astronomical images.
At the heart of this architecture is a dual-phase approach: initially, a convolutional neural network estimates the PSF, which is then used in a modified Richardson-Lucy algorithm to deconvolve the image. The second phase employs another deep CNN, trained to identify and eliminate residual artifacts, ensuring the output image remains as true to the original astronomical object as possible. This is achieved without using oversimplification techniques like Gaussian blur, which can also introduce unwanted effects such as “ringing.”
This model’s significance extends beyond its immediate applications in astrophotography. For edge computing, where processing power and storage capacity are at a premium, the efficiency and effectiveness of this novel CNN architecture promise a new era of high-fidelity imaging. The ability to process and store vast amounts of optical data at the edge opens up new possibilities for research, allowing for real-time analysis and decision-making in observational campaigns across the industry.
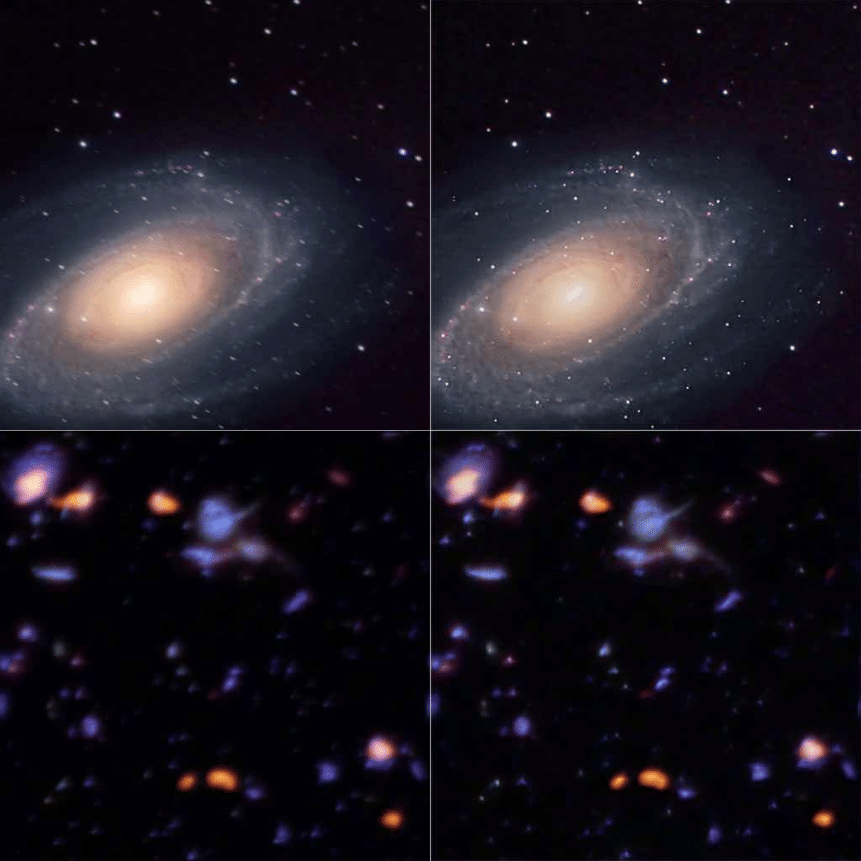
Hubble Legacy Images, Artificial Blur (left), vs. CNN Processing (right)
The advancement in deconvolution techniques undertaken in our lab marks a pivotal moment in imaging of all types. By innovatively leveraging deep learning, we stand on the brink of unlocking the additional potential of a digital image, here demonstrated by capturing the universe with clarity and precision previously reserved for only the highest end of configurations. We have been training this model in our lab for quite some time, so keep an eye out for a full report soon.
What This Means for Astrophotography
The advancement of a novel convolutional neural network (CNN) architecture for astronomical image restoration over traditional deconvolution techniques marks a pivotal development in astrophotography. Unlike conventional methods, which often introduce artifacts like noise and ghost images, the CNN approach minimizes these issues, ensuring clearer and more accurate celestial images.
This technique enhances image clarity and allows for more precise data extraction from astronomical observations. By leveraging deep learning, we significantly improve the fidelity of astrophotography, paving the way for deeper insights into the cosmos with minimal compromise in image processing.
Two Inferencing Use Cases in Edge-Based AI-Accelerated Scientific Research
Data handling and processing methods play pivotal roles in scientific research, particularly in fields requiring extensive data capture and analysis, such as astrophotography. We decided to look at two common inferencing use cases leveraging high-capacity Solidigm storage solutions and advanced computational infrastructure provided by Dell to manage and interpret the vast datasets generated at the edge.
Case 1: Sneaker Net Approach
The Sneaker Net approach is a time-honored method of data transfer that involves capturing data locally on high-capacity storage devices and then physically transporting these storage media to a central data center or processing facility. This method is reminiscent of the early days of computing, where data was moved manually due to the lack of or slow network connections. In edge-based AI-accelerated scientific research, this approach can be beneficial in scenarios where real-time data transmission is hampered by bandwidth limitations or unreliable internet connectivity.
The primary benefit of the Sneaker Net approach lies in its simplicity and reliability. High-capacity SSDs can store massive amounts of data, ensuring that large datasets can be transported safely without continuous internet connectivity. This method is especially advantageous in remote or challenging environments where astrophotography often occurs, such as remote wilderness areas far from conventional internet services.
However, the Sneaker Net approach also has significant limitations. The most obvious is the delay in data processing and analysis, as physical transportation takes time, impeding potential insights that could be derived from the data. There’s also an increased risk of data loss or damage during transportation. Moreover, this method does not leverage the potential for real-time analysis and decision-making that edge computing can provide, potentially missing out on timely insights and interventions.
Case 2: Edge Inferencing
Edge inferencing represents a more modern approach to data handling in scientific research, particularly suited to the needs of AI-accelerated projects. This process involves capturing data in the field and utilizing an edge server equipped with an NVIDIA L4, to execute first-pass inferencing. This method allows for immediate data analysis as it is generated, enabling real-time decision-making and rapid adjustments to data capture strategies based on preliminary findings.
Edge servers are designed to operate in the challenging conditions often encountered in field research, providing the computational power needed for AI and machine learning algorithms right at the data source. This capability is crucial for tasks requiring immediate data analysis, such as identifying specific astronomical phenomena in vast datasets captured during astrophotography sessions.
The advantages of edge inferencing are manifold. It significantly reduces the latency in data processing, allowing for instant insights and adjustments. This real-time analysis can enhance the quality and relevance of data captured, making research efforts more efficient and effective. Edge inferencing also reduces the need for data transmission, conserving bandwidth for essential communications.
However, edge inferencing also poses challenges. The initial setup and maintenance of edge computing infrastructure can be complex and costly, requiring significant investment in hardware and software. There’s also the need for specialized expertise to manage and operate edge computing systems effectively.
Furthermore, while edge inferencing reduces data transmission needs, it still requires a method for long-term data storage and further analysis, necessitating a hybrid approach combining local processing with central data analysis. Thanks to improving compute, storage, and GPU technologies, these challenges are becoming less of an issue.
Both the Sneaker Net approach and edge inferencing offer valuable methods for managing the vast datasets generated in edge-based AI-accelerated scientific research. The choice between these methods depends on the specific requirements of the research project, including the need for real-time analysis, the availability of computational resources in the field, and the logistical considerations of data transportation. As technology advances, the potential for innovative solutions to these challenges promises to further enhance the efficiency and effectiveness of scientific research at the edge.
Extreme Environmental Conditions
In our ever-evolving commitment to pushing the boundaries of technology and understanding its limits, we embarked on a unique testing journey with the Dell PowerEdge XR7620 server and Solidigm QLC SSDs. It’s worth noting that venturing outside the specified operational parameters of any technology is not recommended and can void warranties or, worse, lead to equipment failure. However, for the sake of scientific curiosity and to truly grasp the robustness of our equipment, we proceeded with caution.
Our testing for this project was conducted in the harsh embrace of winter, with temperatures plummeting to -15°C and below amidst a relentless snowstorm. These conditions are far beyond the normal operating environment for most electronic equipment, especially sophisticated server hardware and SSDs designed for data-intensive tasks. The goal was to evaluate the performance and reliability of the server and storage when faced with the extreme cold and moisture that such weather conditions present.
Remarkably, both the server and the SSDs performed without a hitch. There were no adverse effects on their operation, no data corruption, and no hardware malfunctions. This exceptional performance under such testing conditions speaks volumes about these devices’ build quality and resilience. The Dell PowerEdge XR7620, with its ruggedized design, and the Solidigm SSDs, with their advanced technology, proved themselves capable of withstanding environmental stressors that go well beyond the cozy confines of a data center.
While showcasing the durability and reliability of the equipment, this test should not be seen as an endorsement for operating your hardware outside of the recommended specifications. It was a controlled experiment designed to explore the limits of what these devices can handle. Our findings reaffirm the importance of choosing high-quality, durable hardware for critical applications, especially in edge-computing scenarios where conditions can be unpredictable and far from ideal.
Closing Thoughts
We’ve been enamored with high-capacity enterprise SSDs ever since QLC NAND came to market in a meaningful way. Most workloads aren’t as write-intensive as the industry believes; this is even more true regarding data collection at the edge. Edge data collection and inferencing use cases have an entirely different set of challenges.
Like the astrophotography use case we’ve articulated here, they are usually limited in some way compared to what would be found in the data center. As with our research and edge AI effort, the Dell server has just four bays, so the need to maximize those bays to capture our data is critical. Similar to other edge uses we’ve examined, like autonomous driving, the ability to capture more data without stopping is vital.
The conclusion of our exploration into the unique applications of high-capacity enterprise SSDs, particularly of QLC NAND technology, underscores a pivotal shift in how we approach data collection and processing at the edge. The SSDs we used in our tests stand out as particularly interesting due to their capacity and performance metrics, enabling new research possibilities that were previously constrained by storage capabilities.
Our journey through the intricacies of edge data collection and inferencing use cases, encapsulated by the astrophotography project, reveals a nuanced understanding of storage needs beyond the data center. In projects like this, where every byte of data captured, a fragment of the cosmos, has value. Between weather and time constraints, the luxury of expansive storage arrays and racks upon racks of gear is not always available.
This scenario is not unique to astrophotography but is echoed across various edge computing applications and research disciplines. Here, capturing and analyzing vast amounts of data on the fly is paramount. For many industries, interrupting data offloading is a luxury that can neither be afforded nor justified. The SSDs solve this dilemma with their expansive storage capacities. They allow for extended periods of data collection without frequent stops to offload data, thereby ensuring the continuity and integrity of the research process.
These high-capacity SSDs unlock new research frontiers by supporting the data-intensive requirements of AI and machine learning algorithms directly at the edge. This capability is crucial for real-time data processing and inferencing, enabling immediate insights and actions based on the collected data. Whether it’s refining the parameters for data capture based on preliminary analysis or applying complex algorithms to filter through the celestial noise for astronomical discoveries, the role of these SSDs cannot be overstated.
The Solidigm SSDs are not just storage solutions, but enablers of innovation. They represent a leap forward in addressing the unique challenges of edge computing, facilitating research endeavors that push the boundaries of what is possible. The importance of robust, efficient, and high-capacity storage solutions will only grow as we continue to explore the vastness of space and the intricacies of our world through edge-based AI-accelerated scientific research. These technologies do not just support the current research needs; they anticipate the future, laying the groundwork for future discoveries.
This report is sponsored by Solidigm. All views and opinions expressed in this report are based on our unbiased view of the product(s) under consideration.
Engage with StorageReview
Newsletter | YouTube | Podcast iTunes/Spotify | Instagram | Twitter | TikTok | RSS Feed