The Supermicro X13 SuperBlade chassis and GPU blades are a highly adaptable, robust choice, especially for medium-sized AI tasks.
Supermicro has been a pioneer in blade server technology, and its SuperBlade systems serve as a testament to this. The introduction of the Supermicro X13 SuperBlade chassis and blades opens a new chapter for the technology with GPU-enabled blades and the integration of the latest Emerald Rapids CPUs and NVIDIA H100 GPUs. These advancements bring exceptional processing power and efficiency, making the X13 an ideal candidate for various high-end applications.
Design and Specifications
The Supermicro X13 SuperBlade chassis retains the familiar 8U chassis design, known for its high density and flexibility. Each chassis supports up to 20 blades, with the latest offering significantly enhanced by integrating Emerald Rapids CPUs and NVIDIA H100 GPUs. This potent combination promises to deliver unprecedented computational capabilities. Furthermore, the chassis has 200G InfiniBand and 25G Ethernet communications, ensuring high-speed data transfer and networking efficiency.
Popular Use Cases:
- Data Analytics: With the advanced processing power of the Emerald Rapids CPUs and the accelerated computing capabilities of the NVIDIA H100 GPUs, the X13 SuperBlades are exceptionally well-suited for demanding data analytics tasks. These tasks include real-time data processing and extensive data mining operations, which are increasingly critical in today’s data-driven world.
- Artificial Intelligence and Machine Learning: The X13 SuperBlades offer the necessary horsepower for AI and machine learning models, particularly deep learning algorithms requiring substantial computational resources.
- High-Performance Computing: Scientific simulations, medical research, and advanced computational tasks in engineering will benefit significantly from the X13’s enhanced performance, making it a prime choice for high-performance computing applications.
- Cloud Computing: The blades’ increased density and performance make them ideal for cloud service providers. They can handle many cloud-based applications and services, including those that require intensive virtualization and containerization.
- Networking and Communications: Equipped with 200G InfiniBand and 25G Ethernet communications, the X13 excels in high-bandwidth, low-latency applications, making it suitable for demanding networking and communications tasks. Thanks to its external networking, the SuperBlade can act as a hub, providing InfiniBand and Ethernet communications with traditional non-blade servers in the same rack or data center.
In our test rig provided by Supermicro, we had five total blades. Four were equipped with a single processor and the capacity to take a PCIe accelerator, in our case, four NVIDIA H100s and one dual processor blade. We’ll follow up with a subsequent review of the compute blade, the length of this review made its inclusion a little excessive.
Supermicro X13 SuperBlade Data Sheet
Component | Description |
---|---|
Enclosure | 1x SBE-820H2-630 |
PSW | 6x PWS-3K01A-BR |
Fan | 2x PWS-DF006-2F |
BBP | 1x AOC-MB-BBP01-P |
CMM | MBM-CMM-6 |
IB Switch | 1x SBM-IBS-H4020 |
EN Switch | 2x SBM-25G-200 |
Blade Config |
|
Supermicro X13 GPU SuperBlades
The GPU blades at first glance belie their power, with an intake at the front, with our dual processor blade having some 2.5″ NVMe bays in place of the GPU.
Around the back are a dazzling number of pins to connect the blade to the chassis, carrying all the power and data.
Looking inside, we can see the m.2 boot SSDs in the GPU blade.
From above, we can see the air baffling guides. Notice the difference between a GPU blade and a Dual CPU blade. The GPU blade motherboard is identical to the Dual CPU, but just the back I/O half of it.
Around the front, we can start to see the different implementations. The GPU blade has a PCIe riser, while the CPU blade has a U.2 PCIe riser and can accommodate various components in its PCIe slots. The chassis is designed for optimal cooling of passive GPUs by first pulling fresh air into the GPU.
Moving on, starting in the back of the chassis, we can see the PSUs and network connectivity. The top full-width switch is for the 200Gbit NVIDIA Quantum InfiniBand. The larger of the two lower switches is the 25G ethernet, and the small module in the middle is for the Chassis Management Module.
Supermicro X13 SuperBlade Chassis Management and Deployment
Integrating a Chassis Management Module (CMM) into Supermicro’s SuperBlade X13 chassis offers a range of benefits that extend beyond the individual blades to encompass the entire rack, elevating the overall efficiency and manageability of data center operations. The CMM serves as a centralized control point, streamlining the management of the SuperBlade X13 system.
A single pane of glass for all chassis functions is critical for integrated platforms such as a blade chassis. Although the ability to power cycle individual blades might be important to some, a host of other functions play a valuable role in daily management routines.
Supermicro’s CMM offers a central landing spot to monitor the chassis, view the installed blades, and manage the integrated switches installed in the rear of the chassis. This out-of-band management also pulls in device IP addresses, so from that central spot, you can easily hop into each connected device.
Management of each installed blade is similar to that of a standalone Supermicro server. Activities such as BIOS updates are performed through its BMC, as experienced in a previous experiment. This centralized approach enables rapid deployment and consistent updates across all blades, ensuring that each component operates with the latest firmware and settings. Such uniformity is vital in maintaining system stability and performance, especially in dense computing environments where configuration disparities can lead to significant inefficiencies.
The CMM’s role in managing the SuperBlade X13 extends to monitoring and controlling the health of the entire rack. It oversees power consumption, cooling, networking, and system health, providing a holistic view of the rack’s performance. This surveillance is crucial in identifying and addressing potential issues before they escalate, minimizing downtime, and maintaining optimal operational efficiency.
The CMM, in addition to managing the server blades, also handles network management through the same single interface. This allows users to easily access and view the switch management screens of both attached switches, with their respective IP addresses displayed. The CMM can also communicate with neighboring systems for larger deployments, providing a comprehensive management package.
In essence, the CMM transforms the management of the SuperBlade X13 from a series of individual tasks into a cohesive, streamlined process. It’s akin to having a command center that simplifies each blade’s management and enhances the entire rack’s overall performance and reliability. This approach to blade and rack management is helpful to hardware management teams, especially in data centers where scalability, reliability, and efficient use of time are paramount.
Supermicro SuperBlade SBI-411E-5G – NVIDIA H100 Performance
In high-performance computing, the SuperBlade SBI-411E-5G, with an NVIDIA H100, is a versatile and potent tool for distributed training and single-blade inferencing. This flexibility is particularly evident when computational demands fluctuate significantly, such as in data centers managing varying workloads.
Distributed Training Scenarios
The SuperBlade H100 nodes excel in distributed training, a process vital for complex AI models. Imagine a scenario where a large-scale neural network model is being trained on a vast dataset. The model’s training is distributed across multiple blades, each harnessing the power of the H100’s advanced GPUs. This distribution accelerates the training process and allows for handling larger models and impractical datasets on single machines.
The 200G InfiniBand plays a critical role here. Its high-bandwidth, low-latency communication is essential for distributed training, where rapid and efficient data exchange between blades is crucial. This connectivity ensures that the data and learning parameters are consistently and quickly synchronized across all blades, minimizing bottlenecks often encountered in high-volume data processing.
Distributed Training In The Lab
Distributed training has revolutionized how we approach large-scale machine learning and deep learning tasks. Data is king, and the ability to process vast amounts of training data efficiently has been the bottleneck for some time. This is where open-source libraries and powerful hardware, such as the Supermicro SuperBlade X13 with four PCIe GPUs, become game changers, especially when connected through a high-speed 200G InfiniBand network.
Open-source libraries, such as TensorFlow and PyTorch, have become staples in the machine learning community, with support and validation from every manufacturer. They offer robust, flexible, and constantly evolving frameworks for developing and scaling machine learning models. The computational requirement can be staggering when training complex models, such as those used in natural language processing or computer vision. This is where the SuperBlade X13 steps in.
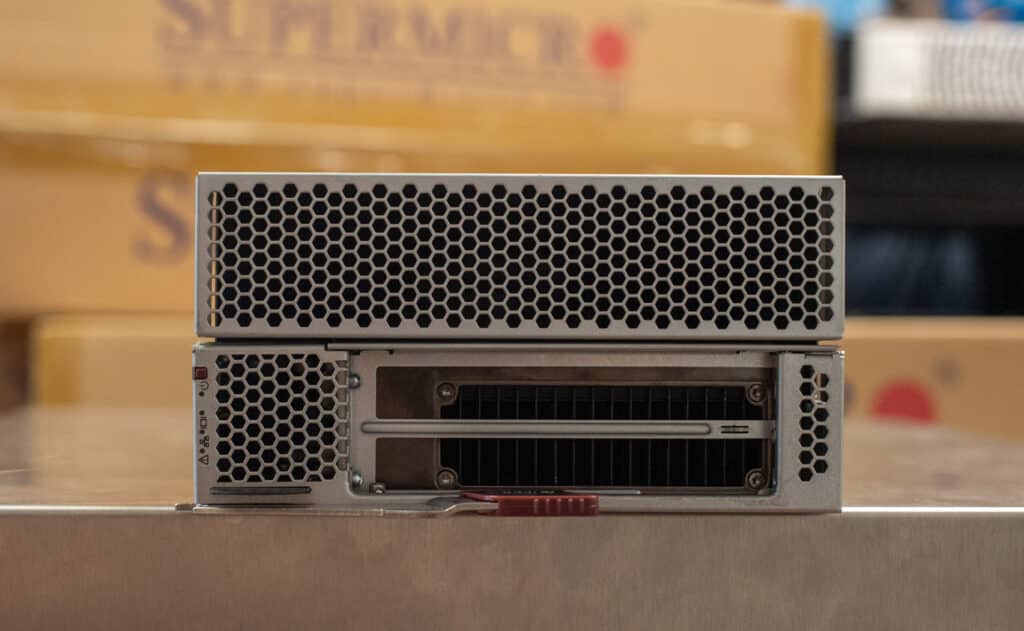
GPU-enabled X13 Blade
The SuperBlade X13 platform is well known for its high-density computing capabilities, making it an excellent choice for HPC environments. Using the double-wide, half-height SBI-411E-5G blades equipped with H100 PCIe GPUs, the SuperBlade X13 supports up to 10 GPUs on air cooling, and up to 20 GPU’s with a liquid cooling per chassis to handle immense parallel processing tasks. Importantly, the blades can be reconfigured at any time really, making them exceedingly flexible as a business’s AI workloads change.
Bringing InfiniBand into the chassis, with extremely low latency and high throughput, helps data and model parameters constantly shuttle between nodes. This high-speed network significantly reduces the data transfer time, often a bottleneck in distributed systems, especially when dealing with large-scale data sets and complex model architectures.
Integrating open-source libraries for distributed training on this setup involved several key steps. First, we had to select optimized containers and libraries to fully utilize GPU capabilities. This consists of using CUDA-enabled versions of these libraries, ensuring they can directly leverage the GPU’s processing power. Second, InfiniBand must be harnessed with NCCL (NVIDIA Collective Communications Library), providing optimized communication routines for collective multi-GPU/multi-node communication.
In practice, when setting up a distributed training task on this platform, each node (in this case, each SuperBlade) runs a part of the model. The model parameters are synchronized across the nodes in real-time, facilitated by the speed and low latency of the InfiniBand network. This synchronization is crucial for the convergence and accuracy of the model.
TensorRT and LLM’s
NVIDIA’s TensorRT Large Language Model (LLM) represents a significant advancement in artificial intelligence and machine learning. Engineered for efficiency and speed, TensorRT LLM is a pivotal component in the ecosystem of Blade server systems, known for its exceptional performance in processing complex AI tasks. Its design caters to the needs of technical professionals and IT decision-makers, offering a robust solution for handling the demanding computational requirements of modern data centers.
The technical framework of NVIDIA’s TensorRT LLM is built to harness the full potential of AI and deep learning. It is designed to optimize neural network inference, making it an ideal choice for high-performance computing environments. The TensorRT LLM achieves remarkable efficiency through its ability to convert trained models into optimized runtime engines, significantly reducing latency and increasing throughput. This feature mainly benefits Blade server systems, where rapid data processing and minimal response times are crucial. Additionally, its compatibility with NVIDIA’s extensive range of GPUs enhances its versatility, making it a scalable solution in varied IT settings.
One of the standout features of NVIDIA’s TensorRT LLM is its capability for distributed training. This aspect is particularly crucial in environments where large-scale machine-learning models are the norm. Distributed training allows TensorRT LLM to leverage multiple systems, distributing the computational load efficiently. This leads to a significant reduction in training time for complex models without compromising on accuracy or performance. The ability to perform distributed training across various nodes makes TensorRT LLM highly adaptable to expansive IT infrastructures, often found in large organizations and research facilities. Furthermore, this distributed approach facilitates the handling of massive datasets, a common challenge in advanced AI projects, thus enabling more robust and sophisticated AI model development.
TensorRT LLM’s optimization and high-performance inference capabilities are ideally suited to the dense, interconnected nature of Blade servers. By leveraging TensorRT LLM, Blade systems can execute complex AI models more efficiently, leading to faster processing times and reduced latency. This is especially critical in scenarios where real-time data analysis and decision-making are essential, such as financial modeling or healthcare diagnostics.
Combining the Supermicro SuperBlade with the distributed training capabilities and adaptability of TensotRT LLM across multiple systems increases the asset’s value for technical professionals and IT decision-makers. By leveraging this powerful combination, organizations can efficiently handle large-scale AI projects, ensuring faster processing, reduced latency, and scalable AI deployments. To facilitate this, we use the Quantum InfiniBand network within the chassis.
Single Blade Inferencing Performance Benchmark with MLPerf
The architecture of one CPU to one GPU per node in the GPU blades offers potential benefits for AI and data analytics workloads, especially for single-blade inferencing tasks. This design provides a balanced ratio of processing power, enabling optimal utilization of the GPU’s capabilities.
To test the Single Blade Inferencing performance, we ran MLPerf 3.1 Inference, both offline and server. BERT (Bidirectional Encoder Representations from Transformers) is a transformer-based model primarily used for natural language processing tasks like question answering, language understanding, and sentence classification. ResNet-50 is a convolutional neural network (CNN) model widely used for image classification tasks. It’s a variant of the ResNet model with 50 layers, known for its deep architecture yet efficient performance.
Single Node Inference | |
---|---|
ResNet-50 – Offline: | 46,326.6 |
ResNet-50 – Server: | 47,717.4 |
BERT K99 – Offline: | 3,702.4 |
BERT K99 – Server: | 4,564.11 |
- Offline Mode: This mode measures a system’s performance when all data is available for processing simultaneously. It’s akin to batch processing, where the system processes a large dataset in a single batch. This mode is crucial for scenarios where latency is not a primary concern, but throughput and efficiency are.
- Server Mode: In contrast, server mode evaluates the system’s performance in a scenario mimicking a real-world server environment, where requests come in one at a time. This mode is latency-sensitive, measuring how quickly the system can respond to each request. It’s crucial for real-time applications where immediate response is necessary, such as in web servers or interactive applications.
In inferencing tasks, the GPU is primarily responsible for the computational heavy lifting. By pairing it with a dedicated CPU, the system ensures that the GPU can operate efficiently without being bottlenecked by shared CPU or platform resources. This is crucial in real-time data processing scenarios like live video analysis or on-the-fly language translation.
Interestingly, we observed that this 1:1 CPU-to-GPU ratio allows for greater predictability in performance. Each node operates independently, ensuring consistent processing times and reducing variability in inferencing tasks. This predictability is vital in environments where response time is critical.
Overall, the one CPU to one GPU configuration in the SuperBlade H100 maximizes the effectiveness of both components. This ensures that each node delivers optimal performance for inferencing tasks, with each node operating independent models and processes. This architecture enhances the system’s ability to handle real-time data processing demands efficiently and reliably.
Adaptive Workload Management
After considering all the information, it is evident that the SuperBlade system is highly adaptable. During peak hours, when the demand for inferencing is high, more GPU-enabled blades can be dynamically allocated to handle these tasks, ensuring efficient handling of real-time requests. Conversely, during off-peak hours, these resources could be shifted to focus on finetuning AI models or processing less time-sensitive tasks. This flexibility allows for optimal utilization of resources, ensuring that the SuperBlade system is robust and efficient in managing varying computational loads.
Benefits of 200G NVIDIA Quantum InfiniBand in These Scenarios
Including 200G InfiniBand in the SuperBlade H100 system enhances these scenarios by providing the backbone for high-speed data transfer. Distributed training enables faster synchronization of data across blades, which is essential for maintaining the consistency and speed of the training process. Single-blade inferencing ensures that large datasets can be quickly moved to the blade for processing, reducing latency and increasing throughput.
What’s Up With Quantum InfiniBand?
InfiniBand, a cornerstone of high-performance computing, is a high-speed interconnect technology initially developed to address the ever-increasing data transfer and communication demands within supercomputing clusters. This highly specialized networking solution has evolved over the years, offering extremely low latency and high bandwidth, making it ideal for connecting servers, storage systems, and other components in HPC environments.
The Supermicro X13 blades we were shipped came equipped with 200G InfiniBand networking and 25G ethernet. This was particularly useful when working on distributed training and other latency and data-intensive tasks. After a few highly variable (and time-consuming) epochs of the training mentioned above, we determined we needed a different metric to provide the InfiniBand network’s real-world test metrics hidden in the blade chassis’s countless pins. With the extreme variability of run-to-run finetuning, it would be irresponsible to try to quantify the impact, or lack thereof, of using a multi-node system like this for these tasks. The results were beyond surprising.
Enter NVIDIA ClusterKit. NVIDIA ClusterKit is a toolkit designed to test the full potential of multinode GPU clusters, offering AI and HPC practitioners an interesting suite of tools to gauge their workloads’ performance, efficiency, and scalability.
We focused on two key tools in ClusterKit:
- Bandwidth Testing: Bandwidth is a critical metric in HPC, reflecting the amount of data that can be transmitted over the network in a given time. We utilized NVIDIA ClusterKit to measure the bidirectional (duplex) bandwidth between nodes in the Supermicro SuperBlade setup. Duplex measurements are essential as they reflect the real-world scenario where data flows simultaneously in both directions.
- Latency Testing: Latency, or the time it takes for a message to travel from one point to another in the network, is another crucial performance metric. Low latency is significant in tightly coupled HPC applications. NVIDIA ClusterKit’s ability to accurately measure duplex latencies provided valuable insights into the responsiveness of the InfiniBand network on the SuperBlades.
SuperBlade InfiniBand and H100 GPU Benchmarking Results with ClusterKit
Going into this section, it is important to understand that each node is identified by a unique tag (e.g., smci-a7, smci-a1, etc.). The denotation of -1, -3, -5, and -7 is the hostname, which reflects the physical position of the blade in the chassis.
The first test focused on measuring the bidirectional bandwidth between various nodes in the cluster. The test involved a message size of 8,388,608 bytes, iterated 16 times.
GPU Direct Tests
Up first, we take a look at the GPU Direct tests. This reports the absolute maximum throughput of the blade platform, utilizing all the latest and greatest SDK’s and toolkits available at the time of writing. It is important to note that the test reports the bandwidth in duplex, meaning the bandwidth is a total in both directions. The single direction would be approximately half. The key takeaway is that the limiting factor for bandwidth is the 200G InfiniBand, but as we will see later, this is not much cause for concern.
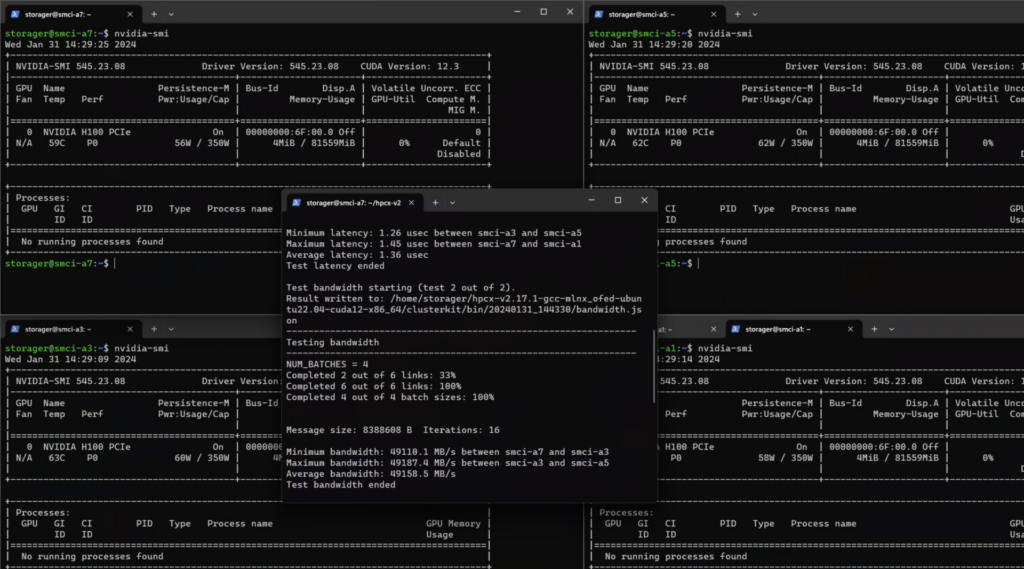
Infiniband ClusterKit Testing on the Supermicro SuperBlades with Divyansh Jain
The matrix below shows the bidirectional bandwidth using GPUDirect.
Bandwidth Matrix MB/s
Rank/Node | smci-a7 | smci-a1 | smci-a3 | smci-a5 |
---|---|---|---|---|
0 (smci-a7) | 0.0 | 49,221.6 | 49,193.6 | 49,223.6 |
1 (smci-a1) | 49,221.6 | 0.0 | 49,219.5 | 49,142.7 |
2 (smci-a3) | 49,193.6 | 49,219.5 | 0.0 | 49,219.7 |
3 (smci-a5) | 49,223.6 | 49,142.7 | 49,219.7 | 0.0 |
Latency uSec
Up next was remarkable latency test results, which measured in microseconds. The GPU Direct tests were just as good as having multiple GPU’s local to a host.
Rank | smci-a7 | smci-a1 | smci-a3 | smci-a5 |
---|---|---|---|---|
0 (smci-a7) | 0.00 | 1.38 | 1.24 | 1.38 |
1 (smci-a1) | 1.38 | 0.00 | 1.25 | 1.36 |
2 (smci-a3) | 1.24 | 1.25 | 0.00 | 1.32 |
3 (smci-a5) | 1.38 | 1.36 | 1.32 | 0.00 |
GPU Neighbor Tests
Moving on to the GPU neighbor tests, Again, bandwidth is reported in duplex, meaning the bandwidth is total in both directions. The single direction would be approximately half. This matrix below shows the bidirectional bandwidth between the H100 cards in each of the four nodes. This is not using the acceleration of the GPUDirect libraries. The denotation of 1, 3, 5, and 7 is the hostname, which reflects the physical position of the blade in the chassis.

SBS-IBS-H4020 HRD InfiniBand Switch
GPU Neighbor Bandwidth (MB/s)
The “GPU Neighbor Bandwidth” test measures the data transfer rate between neighboring GPUs within the same system or node. This metric is crucial for applications requiring frequent data exchanges between GPUs in close proximity, such as multi-GPU parallel processing tasks. The higher the bandwidth, the faster the data transfer, leading to potentially improved performance in GPU-intensive applications.
GPU | Bandwidth (MB/s) |
---|---|
smci-a7 with smci-a1 | 30,653.9 |
smci-a3 with smci-a5 | 30,866.7 |
Average | 30,760.3 |
GPU Memory Bandwidth (MB/s)
The “GPU Memory Bandwidth” test evaluates the rate at which data can be read from or stored in a GPU’s memory by the GPU itself. This bandwidth is a critical performance aspect, particularly for applications that involve large datasets or require high throughput for tasks like image processing, simulations, or deep learning. Higher memory bandwidth indicates a GPU’s better ability to handle large volumes of data efficiently. This test shows us that the X13 Blades have no problem sustaining the H100 GPUs.
GPU | Bandwidth |
---|---|
smci-a7-GPU0 | 55,546.3 |
smci-a1-GPU0 | 55,544.9 |
smci-a3-GPU0 | 55,525.5 |
smci-a5-GPU0 | 55,549.8 |
Average | 55,541.6 |
GPU-to-GPU Bandwidth (MB/s)
This test measures the bidirectional bandwidth between different GPUs. It’s essential for tasks that involve complex computations distributed across multiple GPUs, where the speed of data transfer between the GPUs can significantly impact the overall processing time. High GPU-to-GPU bandwidth is beneficial for accelerating multi-GPU workflows and parallel computing tasks.
GPU | smci-a7 | smci-a1 | smci-a3 | smci-a5 |
---|---|---|---|---|
smci-a7-GPU0 | 0.0 | 30,719.8 | 30,817.7 | 30,823.8 |
smci-a1-GPU0 | 30,719.8 | 0.0 | 30,710.0 | 30,670.9 |
smci-a3-GPU0 | 30,817.7 | 30,710.0 | 0.0 | 30,835.1 |
smci-a5-GPU0 | 30,823.8 | 30,670.9 | 30,835.1 | 0.0 |
Average | 30,762.9 |
GPU0 to Remote Host Bandwidth (MB/s)
The “GPU0 to Remote Host Bandwidth” test quantifies the data transfer rate between the primary GPU (GPU0) and a remote host system. This is vital in distributed computing environments where data needs to be frequently moved between the main GPU and other parts of a networked system, affecting tasks like distributed deep learning training or data analysis on remote servers.
GPU | smci-a7 | smci-a1 | smci-a3 | smci-a5 |
---|---|---|---|---|
smci-a7 | 0.0 | 30,804.3 | 30,753.5 | 30,768.1 |
smci-a1 | 30,804.3 | 0.0 | 30,732.9 | 30,679.7 |
smci-a3 | 30,753.5 | 30,732.9 | 0.0 | 30,970.8 |
smci-a5 | 30,768.1 | 30,679.7 | 30,970.8 | 0.0 |
GPU Neighbor Latency (µsec)
The “GPU Neighbor Latency” test measures the time it takes for a small amount of data to travel from one GPU to its neighboring GPU. Lower latency is desirable, especially in applications requiring real-time data processing or high-speed communication between GPUs, such as real-time rendering or complex scientific simulations.
GPU | Latency |
---|---|
smci-a7 with smci-a1 | 11.03 |
smci-a3 with smci-a5 | 11.01 |
GPU to Remote Host Latency (µsec)
The “GPU0 to Remote Host Latency” test gauges the delay in data communication between the primary GPU (GPU0) and a remote host system. This latency is a critical factor in distributed computing environments, influencing the responsiveness and efficiency of applications that rely on the interaction between a GPU and remote systems, such as cloud-based gaming or remote data processing.
GPU | smci-a7 | smci-a1 | smci-a3 | smci-a5 |
---|---|---|---|---|
smci-a7 | 0.00 | 3.35 | 3.36 | 3.33 |
smci-a1 | 3.35 | 0.00 | 3.41 | 3.37 |
smci-a3 | 3.36 | 3.41 | 0.00 | 3.37 |
smci-a5 | 3.33 | 3.37 | 3.37 | 0.00 |
Average | 3.37 |
The NVIDIA ClusterKit tests revealed impressive performance metrics for the InfiniBand network on the Supermicro SuperBlades. The duplex bandwidth tests revealed high data transfer rates, indicating efficient utilization of InfiniBand’s capabilities. Similarly, the latency tests displayed minimal delays, underscoring the network’s suitability for demanding HPC tasks. This means that this platform performs on par with standalone systems and offers a much higher density of compute and networking, all in a unified solution.
Standalone GPU Server Testing
Next, we moved the 4x NVIDIA H100s into a Supermicro 4U AMD EPYC GPU Server that can support all 4 at the same time, we looked to test the GPU to GPU and latency. It’s critical to understand that we’re just trying to understand the performance profile of the cards in this server, without the cross-blade communications. While this 4U server is flexible in terms of the cards it can support, it doesn’t have the extreme composability the Supermicro X13 SuperBlade Chassis offers. Of course, Supermicro as usual offers a solution for every application, including liquid-cooled socketed GPUs as well.
First up let’s look at the peer-to-peer Bandwidth of the 4 GPUs in one platform.
Write Bandwidth (GB/s) – Unidirectional
GPU | GPU0 | GPU1 | GPU2 | GPU3 |
---|---|---|---|---|
GPU0 | 0.00 | 54.29 | 39.50 | 40.51 |
GPU1 | 54.60 | 0.00 | 40.55 | 40.22 |
GPU2 | 40.60 | 38.73 | 0.00 | 54.03 |
GPU3 | 40.99 | 40.33 | 53.79 | 0.00 |
Read Bandwidth (GB/s) – Unidirectional
GPU | GPU0 | GPU1 | GPU2 | GPU3 |
---|---|---|---|---|
GPU0 | 0.00 | 53.17 | 39.23 | 35.69 |
GPU1 | 53.70 | 0.00 | 36.96 | 41.02 |
GPU2 | 36.28 | 39.88 | 0.00 | 53.32 |
GPU3 | 40.40 | 37.08 | 53.68 | 0.00 |
Important to note here that the GPU0 and GPU1 GPUs are on one NUMA node, and GPU2 and GPU3 are on another NUMA Node. You can clearly see here the impact of going across the NUMA node on performance.
Copy Engine (CE) – Write Latency (us)
Finally, measuring GPU to GPU latency.
GPU | GPU0 | GPU1 | GPU2 | GPU3 |
---|---|---|---|---|
GPU0 | 0.00 | 1.67 | 1.64 | 1.64 |
GPU1 | 1.57 | 0.00 | 1.61 | 1.61 |
GPU2 | 1.66 | 1.69 | 0.00 | 1.65 |
GPU3 | 1.65 | 1.66 | 1.61 | 0.00 |
As expected, moving all of the GPUs into a single platform grants us a 2x on bandwidth vs. the Blade’s 200G IB connections. Bandwidth here may be a consideration for the application, but when talking about latency numbers, working on the order of microseconds, there is not a massive change to report going from an average of 1.6us GPU to GPU while all in one chassis, to only 1.5us in the blades when having to traverse the PCIe Bus, the IB switch and back to the GPU is remarkable. But that is not the full story.
Conclusion
The Supermicro X13 SuperBlade, with its Emerald Rapids CPUs and NVIDIA H100 GPUs, is a welcomed evolution of what blade serves can be. Its capabilities extend across various computationally intensive tasks, making it a versatile and robust solution for industries ranging from data analytics to AI and cloud computing. As the demand for high-performance computing continues to grow, the X13 stands ready to meet these challenges, demonstrating Supermicro’s commitment to innovation and excellence in server technology.
All things considered from testing, we are particularly interested in this platform thanks to its unique and highly adaptable nature from a holistic perspective. It’s important to contextualize the application of the platform.
Imagine a scenario in a research department where you have the Supermicro X13 Blade system in your rack for all of your high-compute horsepower. You can use the centralized management infrastructure built into the platform to not only control the blades and the platform itself but also as a hub for control, networking, and management of other pieces of equipment. Connecting a powerful enough storage server nest to the SuperBlade’s to feed the data-hungry GPUs and you can ingest at line speed all of the bits into your models. In this fictitious scenario, we can have all of our GPUs being utilized by day by different researchers, and then when the time comes, link all of the blades over the InfiniBand and have them work together.
The bandwidth testing of the one-to-one relationship of the CPU to GPU also showed that, given a fully loaded blade chassis, you can outperform a single server with add-in card GPUs with the blade system. With a properly designed distributed training workflow, you could see performance that is essentially as good as, or better than having all of the GPUs in a single node, but now you get a platform that can easily pull double duty, halving the upfront GPU cost. Thanks to the support of the latest CPUs, once implemented, we look forward to moving from HDR InfiniBand to NDR, as that would put the SuperBlades above and beyond the performance you could get in a single GPU server platform.
The Supermicro X13 SuperBlade chassis and GPU blades are a highly adaptable, robust choice for those who have evolving or regularly changing AI needs. Through our extended time with the platform, we encountered needs for DRAM, CPU, and GPU changes, or as it is known in the world of AI, “another day,” all handled by the platform with ease. Overall, the platform is solid and lands as an intriguing and powerful appliance for the AI space without leaving much else to be asked of it. Given the price point of competing systems, if you can take advantage of the flexibility of a blade, this is darn near unbeatable.
Engage with StorageReview
Newsletter | YouTube | Podcast iTunes/Spotify | Instagram | Twitter | TikTok | RSS Feed